AAAI2023 Global Knowledge Tracing Challenge
Winners of the Global Knowledge Tracing Challenge @AAAI2023
- First Prize (AUC = 0.8178): Team KeyCoin@USTC: Shuanghong Shen^{1,2}, Sailan Hu^3, Bihan Xu^{1,2}, Zhenya Huang^{1,2}, Qi Liu^{1,2,4}, Enhong Chen^{1,2} (1 Anhui Province Key Laboratory of Big Data Analysis and Application & School of Data Science, University of Science and Technology of China, 2 State Key Laboratory of Cognitive Intelligence, 3 School of Mathematics and Statistics, Wuhan University, 4 Institute of Artificial Intelligence, Hefei Comprehensive National Science Center) Codes
- Second Prize (AUC = 0.8167): Team LZ-SeqKT: Guimei Liu, Huijing Zhan (Institute for Infocomm Research, A*STAR, Singapore)
- Third Prize (AUC = 0.8166): Team comecomeya: Xing Jiang (Netease) Codes
Leaderboard
Certificates
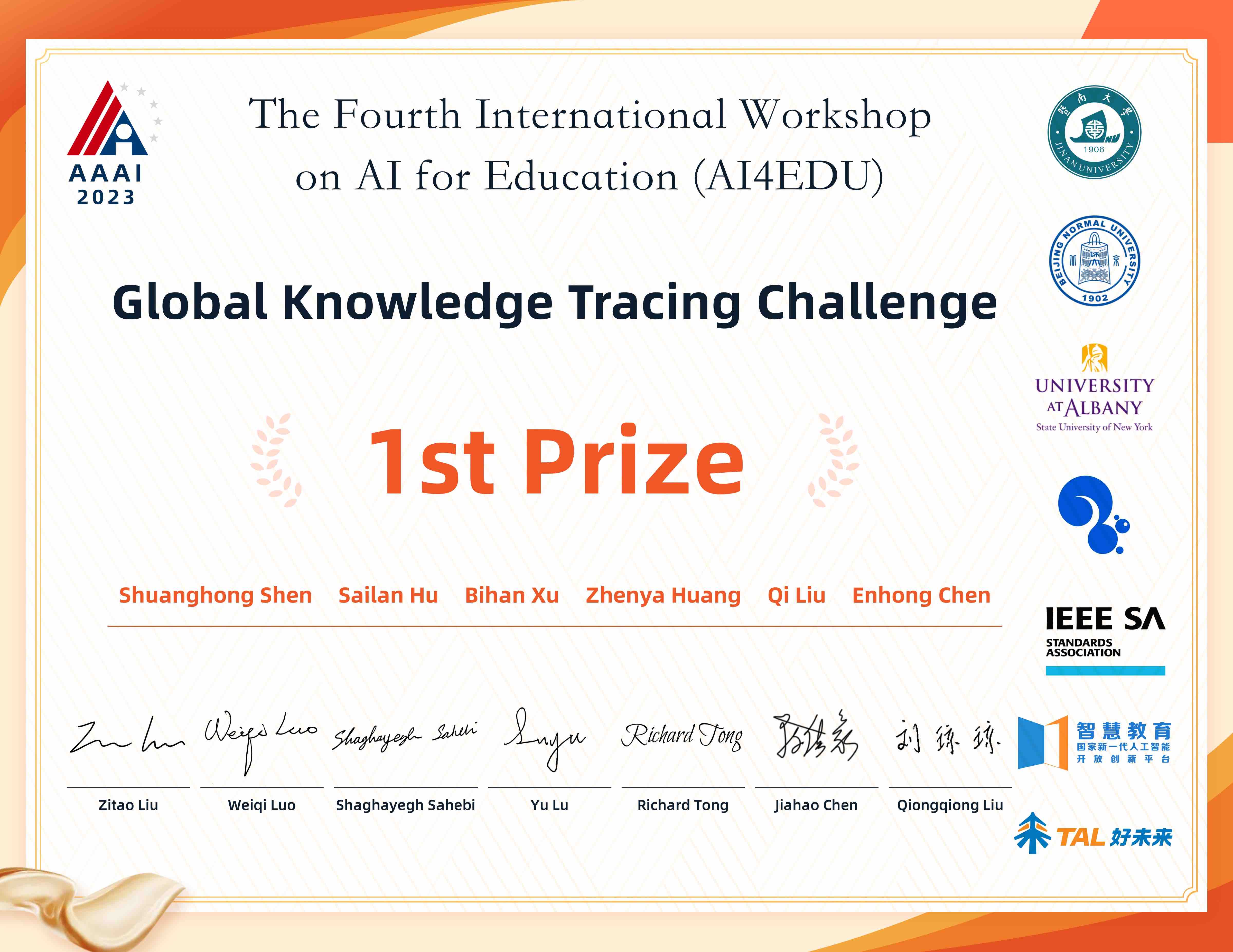
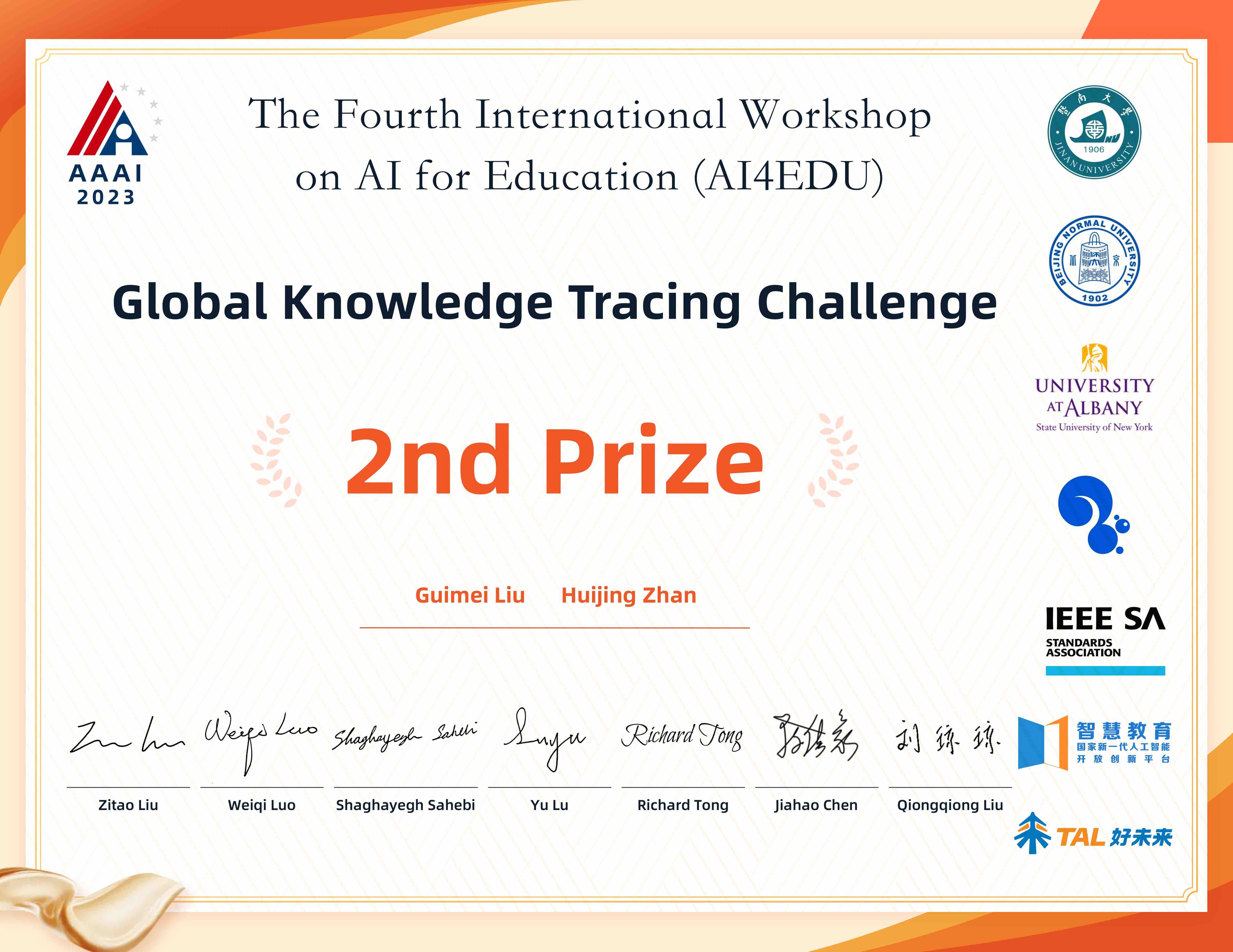
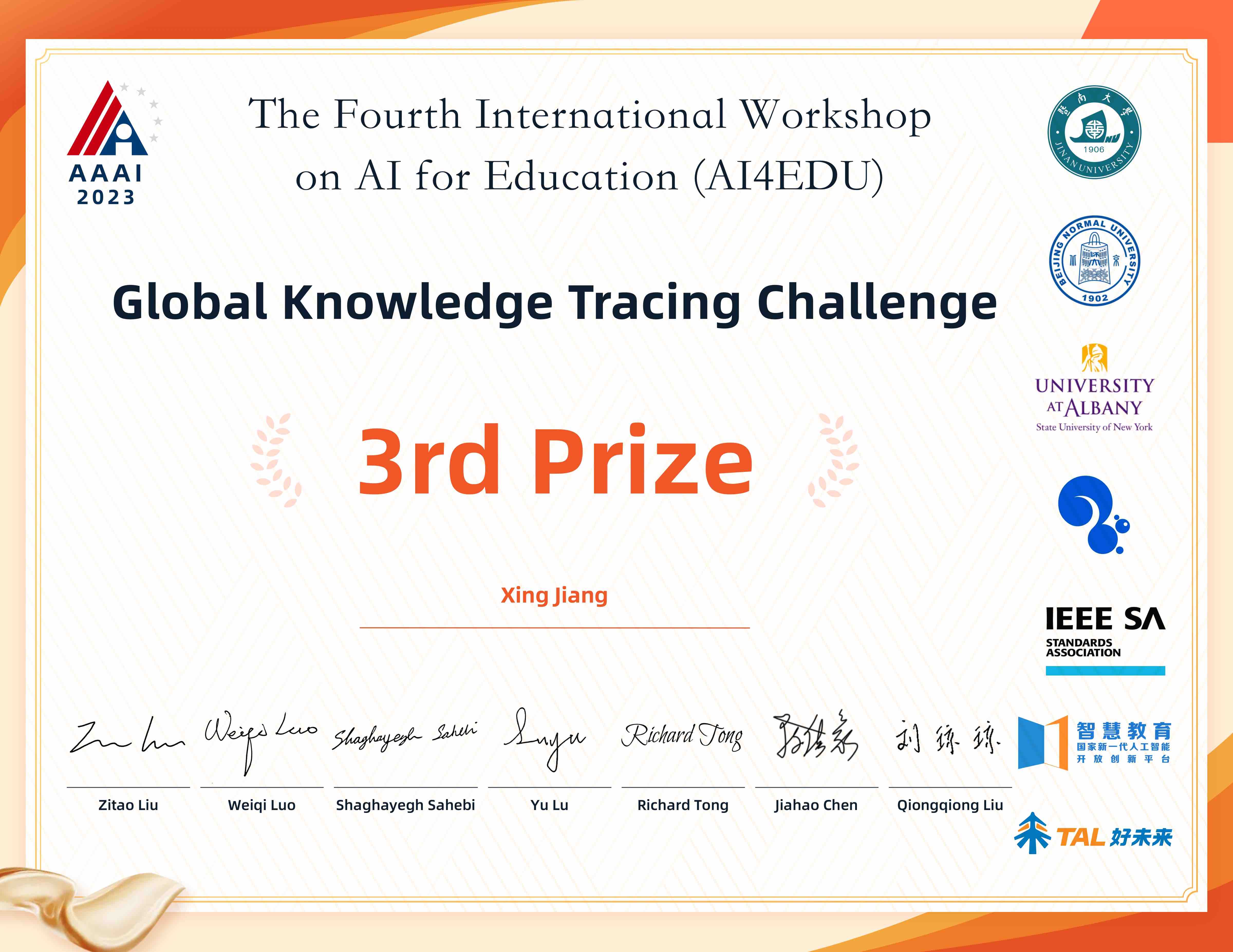
Background
In this competition, we would like to call for researchers and practitioners worldwide to investigate the opportunities of improving the student assessment performance via knowledge tracing approaches with rich side information.
Knowledge tracing (KT) is the task of using students’ historical learning interaction data to model their knowledge mastery over time so as to make predictions on their future interaction performance, shown in the following Figure. Such predictive capabilities can potentially help students learn better and faster when paired with high-quality learning materials and instructions, which is crucial for building next-generation smart and personalized education.
The KT related research has been studied since 1990s where Corbett and Anderson, to the best of our knowledge, were the first to estimate students’ current knowledge with regard to each individual knowledge component (KC). A KC is a description of a mental structure or process that a learner uses, alone or in combination with other KCs, to accomplish steps in a task or a problem. Since then, many attempts have been made to solve the KT problem, such as probabilistic graphical models and factor analysis based models. Recently, due to the rapid advances of deep neural networks, deep learning based knowledge tracing (DLKT) models have become the de facto KT framework for modeling students’ mastery of KCs.
However, even through a large body of deep learning based KT models are proposed, the majority of existing baselines don’t utilize the rich auxiliary side information in educational contexts. Various auxiliary side information could be extracted as external knowledge and integrated with the DLKT models. These auxiliary knowledge are expected to improve DLKT performance, which can be considered as follows:
- Question side information: (1) question text content; (2) latent question variations with respect to each KC; (3) question difficulty level; and (4) relations among questions.
- Student side information: (1) historical successful and failed attempts; (2) recent attempts; (3) students’ learning ability; and (4) individualized prior knowledge of students.
- KC side information: (1) latent knowledge representation; and (2) relations among KCs.
Data & Task Description
We released a large student assessment dataset with rich textual and structural information (well anonymized). The data is collected from students in grade 3 math classes at Xueersi from TAL Education Group that is a leading smart learning solutions provider in China with global footprints. Specifically, the dataset is made up of 18,066 students, 7,652 questions, 1,175 KCs and 5,549,635 interactions/responses. The average student historical sequence length is 307.19. 79.47% responses are with positive labels, i.e., students correctly answer the questions.
Each question is associated with question text content, the explanations of question answers and its corresponding KCs. The relevant KCs are hierarchically structured. All the textual characters are anonymized and mapped into internal unique integer tokens.
Evaluation Task
There are 3,613 student interaction sequences in the test set. For each interaction sequence, the first 50% of interactions are known and the task is to predict the rest 50% interactions’ responses, i.e., 0 or 1.
We will choose to use AUC score as the main evaluation metric for this competition.
Open Sourced Tools
We have re-implemented more than 10 popular deep learning based knowledge tracing models and integrated them into a unified benchmark tool, called pyKT https://www.pykt.org/, including but not limited to:
- DKT: leverages an LSTM layer to encode the student knowledge state to predict the students’ response performances1.
- DKT+: an improved version of DKT to solve the reconstruction and non-consistent prediction problems2.
- DKT-F: an extension of DKT that model the students’ forgetting behaviors3.
- KQN: uses student knowledge state encoder and skill encoder to predict the student response performance via the dot product4.
- DKVMN: designs a static key matrix to store the relations between the different KCs and a dynamic value matrix to update the students’ knowledge state5.
- ATKT: performs adversarial perturbations into student interaction sequence to improve DLKT model’s generalization ability6.
- GKT: utilizes the graph structure to predict the student response performance7.
- AKT: leverages an attention mechanism to characterize the time distance between questions and the past interaction of students8.
- SAKT: utilizes a self-attention mechanism to capture relations between exercises and the student responses9.
- SAINT: uses the Transformer-based encoder-decoder architecture to capture students’ exercise and response sequences10.
Details of pyKT can be found at
Liu, Zitao, et al. “pyKT: A Python Library to Benchmark Deep Learning based Knowledge Tracing Models.” Thirty-sixth Conference on Neural Information Processing Systems Datasets and Benchmarks Track. [PDF]
We highly recommend to utilize pyKT to conduct the KT experiments and build your own KT models upon pyKT. All the provided data files have been processed into the pyKT format and can be fed into pyKT directly.
Data Files
- train_valid_sequences.csv: the main data file to conduct offline model training and validation. Each student interaction sequence at question level is first expanded into KC level when a question is associated with multiple KCs.
Then each sequence is truncated into sub-sequences of length of 200. The data has been split into 5 folds. The file has the following columns:
- fold: the id for each fold, ranging from 0, 1, 2, 3, 4.
- uid: internal user id.
- questions: the sequence of internal question ids.
- concepts: the corresponding sequence of internal KC ids.
- timestamps: regular timestamps.
- selectmasks: if the sub-sequence is less than 200, we add paddings to make it to 200. “-1” indicates these paddings.
- is_repeat: “1” indicates that the current KC and its previous KC belong to the same question.
- pykt_test.csv: the main file for performance evaluation. Each row in pykt_test.csv represents a test student interaction sequence. There are 3,613 student interaction sequences in the test set. For each interaction sequence, the first 50% of interactions are known and the task is to predict the rest 50% interactions’ responses, i.e., 0 or 1. The responses marked as “-1” are those responses to be predicted. Columns of uid, questions, concepts, responses, timestamps, is_repeat are consistent with columns in train_valid_sequences.csv.
Please note that all the question ids and concepts are internal ids mapped and generated by pyKT. They are consecutive integers
-
keyid2idx.json: the id mapping file that stores the id mappings between the original question/KC/user ids and the internal question/KC/user ids generated by pyKT. For example,
"questions": {"355": 0, "1545": 1, "827": 2, "316": 3, "1638": 4, ...
, 355 is the original question id and its corresponding internal question id in pyKT is 0. -
questions.json: the file that contains all the auxiliary information of questions and KCs. The json file structure is defined as follows:
{original question id:
{
"content": the question textual content. The text tokens are anonymized and mapped into internal unique integer tokens.
"concept_routes": all the KCs are stored hierarchically in a tree-like structure. A concept route is a path from the root to the leaf in the KC tree. Different concept routes maybe have the same leaf node, because we build concept paths from different aspects.
"analysis": the textual content of question answer and its detailed analysis. The text tokens are anonymized and mapped into internal unique integer tokens.
"type": the question type. 0: blank filling question; 1: single-choice question; 2: problem-solving question.
}
}
All the data files can be download at https://forms.gle/3TT5VN9ZFnG3JXBPA.
Baseline Results
We have used pyKT to run DKT and AKT on the aforementioned training data and evaluate on our public test sets. All these approaches are purely trained with question/KC ids and student responses without any auxiliary information, such as question content. For the Majority model, we use the correct rate of each question in the training dataset to predict the test set. Details can reference our codes. The results are used as baseline results for this competition:
Model | Non-Accumulative | Accumulative |
---|---|---|
DKT | 0.6801 | 0.7086 |
AKT | 0.7812 | 0.7692 |
Majority | 0.7381 | - |
Practically, there are two different approaches, i.e., accumulative prediction and non-accumulative prediction. The accumulative prediction approach uses the last predicted values for the current prediction while the non-accumulative prediction predicts all future values all at once. Details are discussed in the pyKT paper 11.
Instruction and Codes
We provide easy to use codes and detailed instructions in here.
Discussion Forum
For any question regarding data files and pyKT tools, please post your question at https://github.com/pykt-team/pykt-toolkit/discussions.
Submission Site
We use codalab to receive submissions and please submit your results at https://codalab.lisn.upsaclay.fr/competitions/8087.
Competition Schedule
- November 17, 2022 - Start Date.
- December 31, 2022 - Final submission deadline.
- January 2, 2023 - Final competition results announced.
All deadlines are at 11:59 PM UTC on the corresponding day unless otherwise noted.The competition organizers reserve the right to update the contest timeline if they deem it necessary.
Award
- We will provide cash prizes for the top-3 teams (1st place: $1000; 2nd place: $600 ; 3rd place $300)
- An official certificate will be awarded to the top-3 teams.
- The top-3 teams will be invited to give oral presentations during AAAI 2023.
- The first author of the top-3 teams will be invited to contribute to a competition review paper.
Note: The top-3 teams should make their training and testing code publicly available for verification after the testing submission deadline.
Sponsor
Terms and Conditions
The rules of the competition are as follows:
- Submitted code must use a machine learning model to generate predictions. Submission of hard-coded prediction results is prohibited.
- Users may work in teams of up to 6 people.
- For participants to be eligible for prizes, their code must be publicly available.
Organizers
- Qiongqiong Liu TAL Education Group, China
- Jiahao Chen TAL Education Group, China
- Shuyan Huang TAL Education Group, China
- Zitao Liu TAL Education Group, China
Contact: [email protected]
Reference
-
Piech, Chris, et al. “Deep knowledge tracing.” Advances in neural information processing systems 28 (2015). ↩
-
Yeung, Chun-Kit, and Dit-Yan Yeung. “Addressing two problems in deep knowledge tracing via prediction-consistent regularization.” Proceedings of the Fifth Annual ACM Conference on Learning at Scale. 2018. ↩
-
Nagatani, Koki, et al. “Augmenting knowledge tracing by considering forgetting behavior.” The World Wide Web Conference. 2019. ↩
-
Lee, Jinseok, and Dit-Yan Yeung. “Knowledge query network for knowledge tracing: How knowledge interacts with skills.” Proceedings of the 9th international conference on learning analytics & knowledge. 2019. ↩
-
Zhang, Jiani, et al. “Dynamic key-value memory networks for knowledge tracing.” Proceedings of the 26th international conference on World Wide Web. 2017. ↩
-
Guo, Xiaopeng, et al. “Enhancing Knowledge Tracing via Adversarial Training.” Proceedings of the 29th ACM International Conference on Multimedia. 2021. ↩
-
Nakagawa, Hiromi, Yusuke Iwasawa, and Yutaka Matsuo. “Graph-based knowledge tracing: modeling student proficiency using graph neural network.” 2019 IEEE/WIC/ACM International Conference On Web Intelligence (WI). IEEE, 2019. ↩
-
Ghosh, Aritra, Neil Heffernan, and Andrew S. Lan. “Context-aware attentive knowledge tracing.” Proceedings of the 26th ACM SIGKDD international conference on knowledge discovery & data mining. 2020. ↩
-
Pandey, Shalini, and George Karypis. “A self-attentive model for knowledge tracing.” 12th International Conference on Educational Data Mining, EDM 2019. International Educational Data Mining Society, 2019. ↩
-
Choi, Youngduck, et al. “Towards an appropriate query, key, and value computation for knowledge tracing.” Proceedings of the Seventh ACM Conference on Learning@Scale. 2020. ↩
-
Liu, Zitao, et al. “pyKT: A Python Library to Benchmark Deep Learning based Knowledge Tracing Models.” Thirty-sixth Conference on Neural Information Processing Systems Datasets and Benchmarks Track. ↩